AIJREASVOLUME 6, ISSUE 12aerfpublications2021-12-31T08:33:02+00:00
AIJREAS
VOLUME 6, ISSUE 12 (2021, DECEMBER)
(ISSN-2455-6300) ONLINE
ANVESHANA’S INTERNATIONAL JOURNAL OF RESEARCH IN ENGINEERING AND APPLIED SCIENCES
1.
SELECTION AND APPLICATION OF MACHINE LEARNING- ALGORITHMS FOR ASSESSMENT OF INSTRUMENTATION AND ITS PRODUCTION QUALITY
Mr. E Krishna Mr. B Venkateshwarlu & Dr. S Prasanna
Page 19
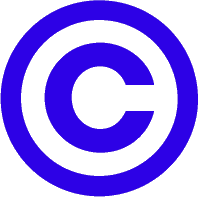 | | | viewed : | 221 Downloads |
2.
A MACHINE LEARNING BASED FRAMEWORK FOR LEVERAGING ANDROID MALWARE DETECTION
Sk.Raheemmujavar & Dr.G.Jaideep
Page 10-16
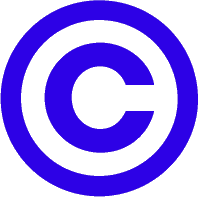 | | | viewed : | 197 Downloads |
3.
A HYBRID APPROACH FOR INTRUSION DETECTION SYSTEM USING K-MEANS AND RANDOM FOREST
Pavan Kumar Suda & Dr.G.Jaideep
Page 17-26
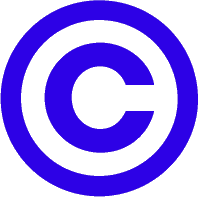 | | | viewed : | 192 Downloads |
4.
ANALYSIS OF GAS TURBINE BLADES THROUGH MODELLING
Shafiuddin Kosgikar & Dr. Mir Safiulla & DR. S. Chakradhar Goud
Page 27-31
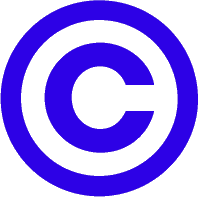 | | | viewed : | 181 Downloads |