AIJREAS VOLUME 9, ISSUE 4 (2024, APR)aerfpublications2024-04-23T15:15:20+00:00
AIJREAS VOLUME 9, ISSUE 4 (2024, APR) (ISSN-2455-6300) ONLINE
ANVESHANA’S INTERNATIONAL JOURNAL OF RESEARCH IN ENGINEERING AND APPLIED SCIENCES
1.
INVESTIGATION STUDY ON IMPROVEMENT IN CBR VALUE OF SOIL REINFORCED WITH JUTE FIBRE
Mr.D.Rambabu, G. Mangireddy, K. Bhanu Sudarshan, P. Venkateshwarao & D.Kollarao
Page 1-10
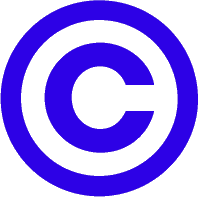 | Paper TitleINVESTIGATION STUDY ON IMPROVEMENT IN CBR VALUE OF SOIL REINFORCED WITH JUTE FIBREAbstractUse of natural fiber in civil engineering for improving soil properties is advantageous because they are cheap, locally available, biodegradable and eco-friendly. The natural fiber reinforcement causes significant improvement in tensile strength, shear strength, and other engineering properties of the soil. Over the last decade the use of randomly distributed natural and synthetic fiber has recorded a tremendous increase.
Keeping this in view an experimental study was conducted on locally available in India, soil reinforced with Jute fiber. In this study the soil samples were prepared at its maximum dry density corresponding to its optimum moisture content in the CBR mould with and without reinforcement. The percentage of Jute fiber by dry weight of soil was taken as 0.25%, 0.5%, 0.75% , 1% and 1.25% In the present investigation the lengths of fiber was taken as 50 mm, 75 mm, 100 mm, 125 mm and 150 mm with diameter of 2 mm were considered for each fiber length.
The laboratory CBR values of soil and soil reinforced with Jute fiber were determined. The effects of lengths and diameters of fiber on CBR value of soil were also investigated. Tests result indicates that CBR value of soil increases with the increase in fiber content. It was also observed that increasing the length and diameter of fiber further increases the CBR value of reinforced soil and this increase is substantial at fiber content of 1.25 % for 150 mm fiber length having diameter 2 mm.
KEYWORDS :
| | viewed : | 46 Downloads |
2.
A COMPARATIVE STUDY OF MATHEMATICAL MODELS FOR RANDOM DATA ANALYSIS: INSIGHTS FROM CASE STUDIES
T. BHOJADAS DR. PRABHA SUDHIR RASTOGI Dr.TVA PADMANABHA SASTRY
Page 11-18
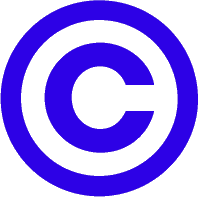 | Paper Title A COMPARATIVE STUDY OF MATHEMATICAL MODELS FOR RANDOM DATA ANALYSIS: INSIGHTS FROM CASE STUDIESAbstractMathematical modeling studies are increasingly recognized as an important tool for evidence synthesis and to inform clinical and public health decision‐making, particularly when data from systematic reviews of primary studies do not adequately answer a research question. The use of a common terminology for modeling studies across different clinical and epidemiological research fields that span infectious and non‐communicable diseases will help systematic reviewers and guideline developers with the understanding, characterization, comparison, and use of mathematical modeling studies. Scientific data is often analyzed in the context of domain-specific problems, for example, failure diagnostics, predictive analysis, and computational estimation. These problems can be solved using approaches such as mathematical models or heuristic methods. In this study we compare a heuristic approach based on mining stored data with a mathematical approach based on applying state-of-the-art formulae to solve an estimation problem. However, systematic reviewers and guideline developers may struggle with using the results of modeling studies, because, at least in part, of the lack of a common understanding of concepts and terminology between evidence synthesis experts and mathematical modellers. The goal is to estimate results of scientific experiments given their input conditions.
KEYWORDS : Mathematical modeling, scientific data, failure diagnostics, predictive analysis, mathematical modellers, input conditions
| | viewed : | 47 Downloads |
3.
STRENGTH OF CONCRETE ON REPLACEMENT OF SAND WITH QUARRY STONE DUST AS FINE AGGREGATE
Mr. K. ANIL, K.Prasanna, M. Pavani, K. Veerraju, S.Vamsi & I. Malyadri
Page 19-28
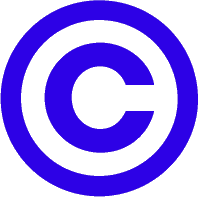 | Paper TitleSTRENGTH OF CONCRETE ON REPLACEMENT OF SAND WITH QUARRY STONE DUST AS FINE AGGREGATEAbstractCommon river sand is expensive due to excessive cost of transportation from natural sources. Also large-scale depletion of these sources creates environmental problems. As environmental transportation and other constraints make the availability and use of river sand less attractive, a substitute or replacement product for concrete industry needs to be found. River sand is most commonly used fine aggregate in the production of concrete poses the problem of acute shortage in many areas.
This Thesis presents the feasibility of the usage of Quarry Rock Dust as 100% substitutes for Natural Sand in concrete. Mix design has been developed for M25 and M40 grades using design approach IS for both conventional concrete and quarry dust concrete. Tests were conducted on cubes and beams to study the strength of concrete made of Quarry Rock Dust and the results were compared with the Natural Sand Concrete. It is found that the compressive and flexural strength of concrete made of Quarry Rock Dust are nearly 10% more than the conventional concrete.
The results show that at a dosage 1.3% of super plasticizer by weight of cement the concrete made of quarry stone dust as fine aggregate attained low strengths when compared with other dosages (1% and 1.6%) in compression and flexure.
KEYWORDS :
| | viewed : | 39 Downloads |
4.
INVESTIGATING CONSUMER PERCEPTION AND ACCEPTANCE OF BIODEGRADABLE PLASTIC PACKAGING IN THE FOOD SECTOR
Arun Kumar Budda Dr. Bhupesh Kumar Sharma Dr. Tumati Srinivasarao
Page 29-36
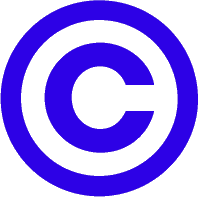 | Paper TitleINVESTIGATING CONSUMER PERCEPTION AND ACCEPTANCE OF BIODEGRADABLE PLASTIC PACKAGING IN THE FOOD SECTORAbstractThis manuscript concerned customer recognition of biodegradable packaging. The authors of this study took up this issue due to its topicality and importance for enterprises and customers. The demand to develop and produce eco-friendly alternatives for food packaging is increasing. The huge negative impact that the disposal of so-called “single-use plastics” has on the environment is propelling the market to search for new solutions, and requires initiatives to drive faster responses from the scientific community, the industry, and governmental bodies for the adoption and implementation of new materials. It explored the role of these packages in marketing activities in the organic products market. Bioplastics are an alternative group of materials that are partly or entirely produced from renewable sources. Some bioplastics are biodegradable or even compostable under the right conditions. Providing correct answers on this topic did not depend on gender, health status, or place of residence, only on the age and education of respondents. The research results may have both practical and theoretical implications. The results contribute to the development of management sciences. The conclusions from the manuscript allow producers, not only in food, to design new, biodegradable packaging in accordance with the feelings and expectations of consumers.
KEYWORDS : Biodegradable packaging, bioplastics, “single-use plastics”, organic products market, education of respondents.
| | viewed : | 50 Downloads |
5.
MATHEMATICAL MODELING FOR RESOURCE ALLOCATION AND DATABASE MANAGEMENT IN CLOUD ENVIRONMENTS
SIDDU RAJU G DR. VISHWAJEET S. GOSWAMI Dr.TVA PADMANABHA
Page 37-43
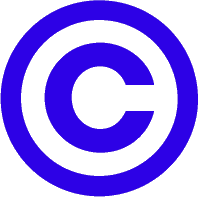 | Paper TitleMATHEMATICAL MODELING FOR RESOURCE ALLOCATION AND DATABASE MANAGEMENT IN CLOUD ENVIRONMENTSAbstractThe primary objective of this research is to devise mathematical frameworks that can effectively allocate computational resources, storage, and network bandwidth to database services in the cloud. The study considers various factors such as workload variations, data access patterns, and performance metrics to create models that adapt dynamically to changing conditions. The mathematical models proposed in this study incorporate optimization algorithms, machine learning techniques, and statistical analysis to predict resource requirements based on historical data and real-time monitoring. By considering the unique characteristics of database workloads, the models aim to strike a balance between performance, cost, and resource utilization. Furthermore, the research investigates the impact of different database management systems (DBMS) on the efficacy of resource allocation models. It explores the challenges and opportunities presented by diverse database technologies and architectures within cloud environments.
KEYWORDS : mathematical frameworks, allocate computational resources, mathematical models, allocation models, cloud environments.
| | viewed : | 43 Downloads |
6.
INVESTIGATION OF IONIC MOBILITY AND STRUCTURAL DYNAMICS IN CONDUCTING GLASSES
P RAJI REDDY DR. GANGA DHAR PAWAR DR. SUDHIR BAIJNATH OJHA
Page 44-51
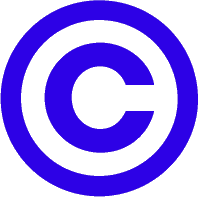 | Paper TitleINVESTIGATION OF IONIC MOBILITY AND STRUCTURAL DYNAMICS IN CONDUCTING GLASSESAbstractThe goal of this research is to shed light on a family of ion-conducting glasses by analyzing their elastic characteristics and structural characteristics. Glasses that carry ions are essential to the operation of many modern systems. S.S. batteries, fuel cells, and sensors all fall under this category. The bond valence method has been applied to reverse Monte Carlo (RMC) produced structural models of a wide range of ion conducting glasses in order to elucidate the relation between the microscopic structure and the ionic conductivity. Our approach allows us to predict the ionic conductivity of the glasses directly from the “pathway volume” of the structural models and to investigate the nature of these low-dimensional conduction pathways. A comp-rehensive structural analysis is carried out in this study using cutting-edge instruments such as X-ray diffraction (XRD), electron microscopy, and spectroscopy. Using these techniques on ion-conducting glasses might provide insight on their overall geometry and atomic composition. Improving the existing performance of energy storage and sensing materials and developing new ones calls for an in-depth knowledge of their structural composition and mechanical behavior. Glassy ionic conductors have attained considerable importance in the solid state battery technology field. Solid state NMR spectroscopy is one of the most powerful tools for addressing these questions.
KEYWORDS : Reverse Monte Carlo (RMC), fuel cells, X-ray diffraction (XRD), Glassy ionic, “pathway volume”.
| | viewed : | 47 Downloads |
7.
A COMPREHENSIVE STUDY ON THE IMPACT OF SALT ON SUNFLOWER GROWTH: BIOCHEMICAL ALTERATIONS IN SEEDING AND EARLY GROWTH STAGES
Sangi Godavari Dr. Dinesh Kumar Singh & Dr.Lyada Srinivas
Page 52-59
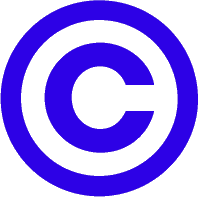 | Paper TitleA COMPREHENSIVE STUDY ON THE IMPACT OF SALT ON SUNFLOWER GROWTH: BIOCHEMICAL ALTERATIONS IN SEEDING AND EARLY GROWTH STAGESAbstractSunflower plants are semi-tolerant to salt stress. Calcium modulates the expression of oubain-sensitive ATPases, responsible for sodium fluxes in cells. Salt stress delays degradation of oil body (OB) membrane proteins. Serotonin and melatonin contents are elevated in response to salt stress. NO negatively regulates the total glutathione homeostasis and regulates polyamine and glycine betaine homeostasis in response to salt stress. An intricate biochemical crosstalk is thus observed to control salt tolerance mechanisms in sunflower. Climate change is causing soil salinization, resulting in crop losses throughout the world. The ability of plants to tolerate salt stress is determined by multiple biochemical and molecular pathways. Here we discuss physiological, biochemical, and cellular modulations in plants in response to salt stress. Accelerated antioxidant activities and osmotic adjustment by the formation of organic and inorganic osmolytes are significant and effective salinity tolerance mechanisms for crop plants. In addition, polyamines improve salt tolerance by regulating various physiological mechanisms, including rhizogenesis, somatic embryogenesis, maintenance of cell pH, and ionic homeostasis. KEYWORDS : Sunflower plants, salt tolerance, organic and inorganic osmolytes, multiple bio-chemical, Salt stress, Sunflower oil, maintenance of cell pH.
| | viewed : | 36 Downloads |
8.
EXPLORING MATHEMATICAL APPLICATIONS OF NON-NEWTONIAN FLUID BEHAVIOR IN PIPELINE SYSTEMS
J Pashupathi Sharma Dr. Vineeta Basotia Dr.TVA Padmanabha Sastry
Page 60-66
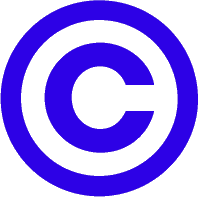 | Paper TitleEXPLORING MATHEMATICAL APPLICATIONS OF NON-NEWTONIAN FLUID BEHAVIOR IN PIPELINE SYSTEMSAbstractThis work explores mathematical models and practical applications of non-Newtonian fluid characteristics, which may have implications for pipeline transport. Most fluid transport systems assume that fluids behave according to Newton\'s laws. There are, however, cases when non-Newtonian fluid characteristics, including shear-thinning or shear-thickening behavior, are necessary. Investigating and using these unique features may lead to more efficient pipeline systems.
In order to illustrate the flow behavior of non-Newtonian fluids, the mathematical models used in the study take rheological aspects and fluid parameters into consideration. Computer fluid dynamics (CFD) models are used to examine the energy requirements, pressure drops, and transport dynamics of non-Newtonian fluids in pipelines. In order to improve the efficiency, effectiveness, and reliability of fluid transfer in pipelines, this research aims to discover solutions that consider the fluid\'s rheological qualities.
The results could have far-reaching consequences as they provide suggestions for the administration and development of pipeline networks that might carry fluids that do not adhere to Newton\'s laws.
KEYWORDS : mathematical models, practical applications, non-Newtonian fluid, pipeline systems, Computer fluid dynamics (CFD).
| | viewed : | 35 Downloads |